Vrinda Kapoor, CEO, 114ai
There are prevalent assumptions about AI – that you need to be data scientist or engineer, a ‘boffin’ in order to understand what is happening ‘within the black box’. And so without these prerequisite skills you should not try to get involved. But this presumption by definition excludes the vast majority of the population, crucially women and people of diverse backgrounds and perspectives, from the discussion about AI and the how and where humanity wants it to be deployed This frightens people into thinking that AI enabled machines will take over their jobs and their lives.1 From this lack of understanding comes a lack of trust, which undermines the potential of this wonderful technology to address many of the issues facing mankind today.
Looking inside the black box
I would like to start by clarifying that the entire perception of AI is actually wrong. AI is not, or shouldn’t be, a black box closed to the majority of the population, leaving them wondering if we are going to end up in some kind of a terminator movie. There are many opportunities for people of all backgrounds and abilities, in particular women or people from all ethnic and social backgrounds to become involved in driving the future of AI in a direction that does not diminish our humankind. The fact is that in the years to come, AI will become an all-pervasive technology that will be embedded in our daily lives, making it all the more important for a wide range of people to really understand the technology- what it is capable of, where it can have touch points in our lives in the future and most importantly, what risks will come along with it.
So far, an understanding of AI has been limited to an elite club of data scientists, mathematicians and coders, leaving a majority of the population out of the conversation. Thus, the question beckons, what skills and abilities does someone need to understand AI or even to build a career in AI? Taking me as an example, I am not a data scientist, or a coder. But I do have the ability to reason, to infer and to deduct, which are the key skills that people need to really identify with AI. I perceive the world around me, comprehend the complexity of the processes that make up our daily lives, and can break that down to understand how these machines can work and imagine what the impact to our society can be.
What is machine cognition?
Machine cognition is really a process of separating background and foreground. It is no longer sufficient to think in terms of machine cognition as merely ‘connecting the dots’. We need to move a step further to a paradigm where machines are able to actually ‘find the dots’ amongst a sea of data in a complex world. The Dual Process Theory is described by cognitive scientists as the bed rock of human cognition. It proposes that the brain thinks in two ways:
- Associative thinking – unconscious (not deliberate) and is believed to organize sensory inputs into similar categories popularly known as pattern recognition (similar to pattern recognition or machine learning); and
- Directed or relational thinking – conscious and directed towards a goal (similar to learning and reasoning).
Proponents of the dual process theory conjecture that the brain has different mechanisms for associative and relational thinking. They argue that critical thinking, creative thinking, brainstorming and problem solving cannot be modelled as simple associative thinking or pattern recognition problems.
AI encompasses both – picking up patterns in high volumes of data but also being able to understand, infer and contextualise high veracity and high variability within that data, the same way a human being does. It is only by applying both associative and relational thinking that machines will be able to have a complete analysis of the whole picture and provide meaningful results.
This is the space that 114ai works in, and just for context, both I as the CEO, as well as the CTO, are women.
Towards a human-machine team
Smart and autonomous machines of the future will require a method to mimic human intelligence in decision-making. The first step in this journey is to get to a state where human and machine teaming is a reality.
In the first step, machines will do what they do best, which is to sift through petabytes of data and bring to the forefront critical information. There is also a parallel need to capture and codify expert knowledge, giving the machine the “intelligence” it needs to reason through these complex pathways. Then, analytics will be built on symbolic and sub-symbolic processing, which includes things like common sense reasoning, background knowledge, episodic memory and institutional memory. The cues from the sub-symbolic process will need to be dis-ambiguated using reasoning techniques.
And finally, for true cognition to be achieved, be successful and viable, we will need to build trust with those machines and understand the factors contributing to a decision-making process.
As the technology matures, AI will be used to provide decision support to assist doctors, pharmacologists, space operators, researchers, governments, and the military. AI will provide tools to assist these and other critical decision makers to leverage the tremendous amount of data being thrown at them constantly, to reduce their cognitive burden and make better decisions. By focussing on provenance, trackability and explainability, we can move away from the current state of ‘black box’ decision making, to a world where we can begin to trust the decisions coming from these machines. This is a necessary technological obstacle we have to overcome before we can even think about having autonomous machines in our homes and on our streets.
Questions for next generation AI
The next generation of AI is going to require us to find answers to a few key questions:
- How can we reduce the friction of adoption? Can we stretch the boundary of what can be achieved in a low code or a no code environment, and drive even bigger value from AI?
- Can we enable machine to machine hyper automation or redirect the limited number of data scientists in the workforce away from mundane tasks towards the more complex problems at the top of the value chain?
- Can we create an operating system for human machine teaming for human beings to interact with and work seamlessly with machines?
- How can we enable augmented intelligence, or create methodologies for machines to think, reason, infer and apply logic to enhance their productivity and more complex operations?
- Can we build an AI paradigm that inspires trust, by establishing those cognitive pathways to maximise trust and to show the provenance of decisions?
Value of a no-code platform
At 114ai we are building a platform that will enable us to leverage data analytic tools and expert knowledge in order to get to cognition or augmented intelligence. The tool we are building is a no-code environment which acts as the ‘glue’ or the information architecture to fuse data, automate data transference, capture expert knowledge, set up those analytic pipelines, and use logical inference to enable the extraction of causal inferences from unstructured data.
The entire pipeline uses innovative knowledge graphs, providing explanations for each step of the inferencing process with a design that enables transparency and builds trust. Our goal is to break down the barriers that enable fear of AI; we want to reduce the friction on uptake and utilisation, enable the development and scale of analytics pipelines and use data to drive decision-making in a no-code environment.
Unlocking the potential of AI
Our vision is to make AI ubiquitous in decision-making. This would unlock tremendous potential across the economy, but it would also allow a focus on areas of research that are not well funded, because they are not considered of commercial value. For example, an area that is close to my heart is the repurposing of existing drugs to treat debilitating conditions which may not make financial sense.
These technologies have long been touted for their promise to make new drugs cheaper and more effective.
The development of tools that can reduce the cost associated with trial and error, narrow down the list of candidate therapies and reduce failure rates between phase 1 trials and regulatory approvals, would have a substantial impact on some of the lower hanging and often neglected therapeutic areas.
This is but one example of where enhanced AI, based on a human-machine team approach, can be deployed to bring about tremendous benefits to mankind. Let us work together to make this dream a reality.
1 This article is based on Vrinda’s presentation to l’Institut EuropIA https://www.youtube.com/watch?v=vfSEBve6MW4&list=PL138pGCdJ57XtKZAY iJ4-SpgYL3pCaXwz&index=8
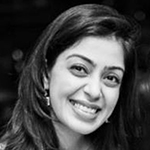
114ai is a data infrastructure company, which builds tools to make AI more accessible and usable. The company was established in 2019, after Vrinda experienced the use of AI enabled technology in the healthcare sector. With a background in life sciences and healthcare, rather than computer science, Vrinda became increasingly interested in the digital transformation of patient management systems, in both the public and private hospital systems in India. From there she became interested in addressing the challenges of AI systems, which were preventing the promise of AI from being realised. She hopes that enabling more women to work in AI related areas will normalise the involvement of women in this specialised area. “I would like to see the industry make an effort to inform young women graduates about the full range of work opportunities that are out there for them – not just those who study computer science, or even STEM, but women from all the liberal arts”, adds Vrinda. Of the challenges facing us over the next ten or so years, Vrinda sees cybersecurity as a major one, especially for individuals who tend not to understand how vulnerable they may be, as smart devices are brought into the home environment, especially around children. “I would like to see senior leaders in the industry, including the GTWN members, working together on making AI more understandable and building back trust with the community.